
Artificial Intelligence: Trade-offs in the Pursuit of High-Quality Data
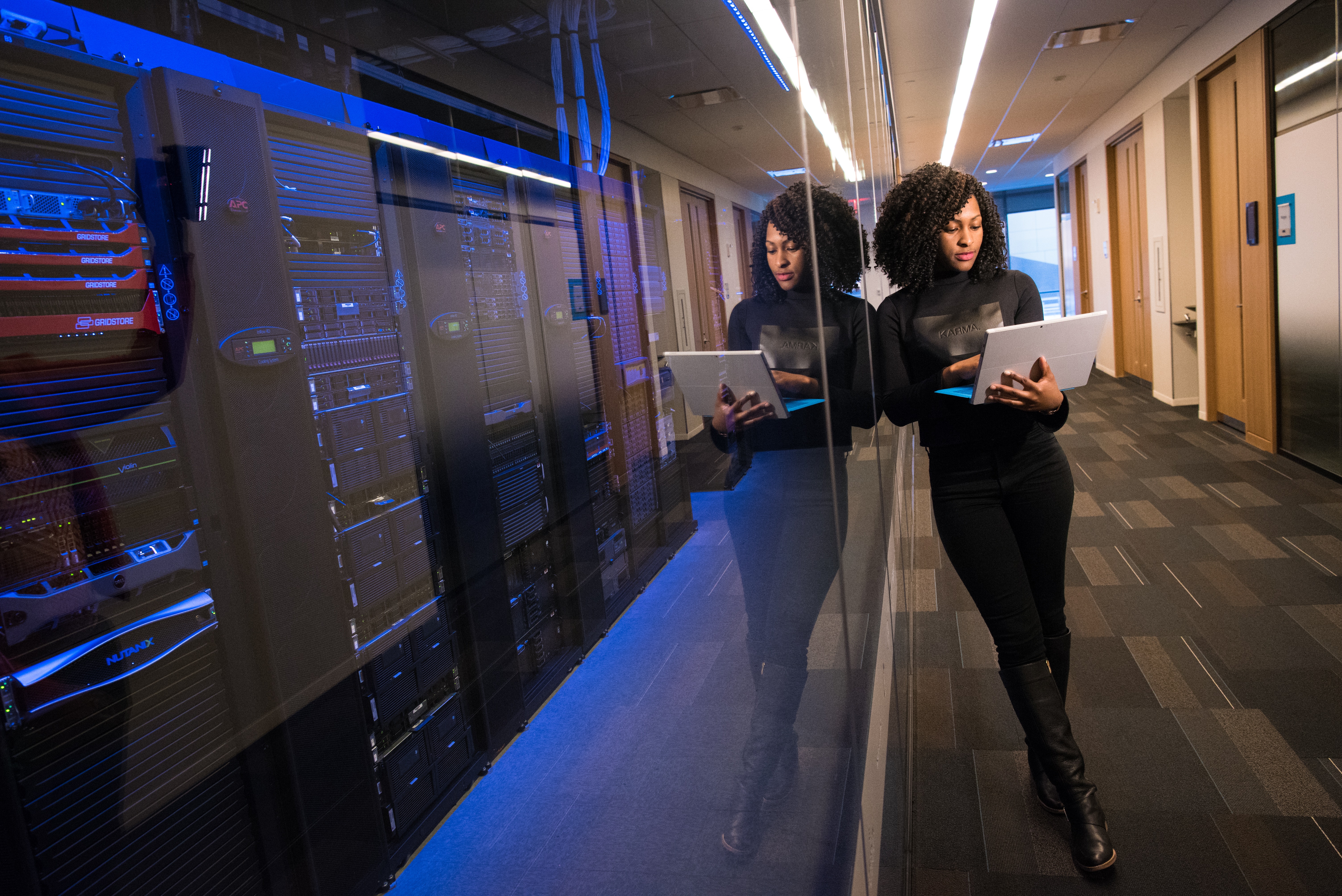
Artificial intelligence (AI) promises to revolutionize the way organizations conduct business. Although the private sector has perhaps embraced this promise most quickly and thoroughly, AI adoption grew steadily across the U.S. government. Besides generating efficiencies that will save taxpayer dollars, AI holds the potential to streamline bureaucratic processes, contributing to a more nimble and responsive government but also to a federal workforce that can apply its talent to creative rather than repetitive tasks.
Fiscal Service launched a four-month study last October to assess whether AI capabilities, like machine learning and natural language processing, could be used to create Treasury warrants. A cross-functional team is evaluating whether it is possible to train a computer algorithm to interpret legislation and correctly identify the three primary parts of a warrant: purpose, dollar amount, and period of availability.
Warrants, which are appropriations orders issued by the US Department of the Treasury (Treasury), play a quiet but important role in the funding of federal agencies. Experienced accountants in Fiscal Service create warrants through a manual process of reviewing published appropriations bills, interpreting the legislation, and identifying the right Treasury accounts. Given the manual process of creating warrants, it presents a clear opportunity for improvement through automation.
As the team makes exciting progress in developing an AI solution, it encountered challenges that required weighing priorities and making trade-offs. These decisions show the realities of pursuing AI in a time-constrained proof of concept.
Creating and training an AI solution requires enough volume of high-quality, well-managed data. The team encountered its first challenge on discovering that machine readable data (i.e. information in a format that a computer can easily consume and understand) was not as readily available as assumed. When signed, appropriations bills are first only published as PDFs rather than in HTML or XML formats, which are published weeks later.
Both HTML and XML files are superior to PDFs from the perspective of extracting data, with fewer downstream formatting issues that need to be cleaned up. Further, XML content can be tagged or labelled with data elements such as bill headings and legislation page numbers that ease classifying the data and subsequent matching to Treasury accounts.
Warrant creation is time sensitive as authority must promptly be recorded for agencies. The Bureau, therefore, does not have the luxury of waiting weeks for the publishing HTML and XML versions. Recognizing this reality, the team decided to trade the cleaner readability of HTML and XML for the immediate availability of PDFs. This decision prioritized developing a solution responsive to a real-world problem over an ideal AI model that exists in a vacuum.
Choosing to use PDFs required the team to devote time in a fast-paced project to cleaning up and organizing extracted data. This included addressing formatting errors such as missing spacing and breaking the data down into components such as agency authority, dates, dollar amounts, and page numbers.
The team then confronted a data management issue when it discovered that the database housing Treasury account data contained irregularities that could confuse the AI model. The team determined it could not validate the data in the time available and, therefore, selected an alternative source of Treasury account data. Unlike the first database, this second source is a static PDF that is only updated quarterly.
Projects involve trade-offs and compromises, and the AI warrants project is no different. The team has weighed expediency against effectiveness, quality against speed, and practicality against ideality. Through all these decisions, the team has focused on how to best address automating a manual process rather than on the novelty of AI technologies.
Although AI promises to improve the federal government’s financial management processes, the technologies require a solid foundation of mature data management practices. Strengthening data quality and preparing the government’s vast volumes of information for consumption will accelerate adopting more innovative practices.
Stay tuned for another update on the AI warrants project and check back at https://www.fiscal.treasury.gov/fit/ as the team evaluates AI methodologies and identifies the most appropriate solution for automating creating Treasury warrants.